Satellite models to strike a pose for competing AIs
Movie special effects fans like to debate CGI versus traditional model effects – an issue about which competing AIs will soon gain direct experience. Trained upon computer-generated images of satellites, AIs will go on to judge the position and orientation of realistic mockups snapped in space-like lighting conditions.
This new machine learning competition is being overseen by ESA’s Advanced Concepts Team and Stanford University’s Space Rendezvous Laboratory (SLAB).
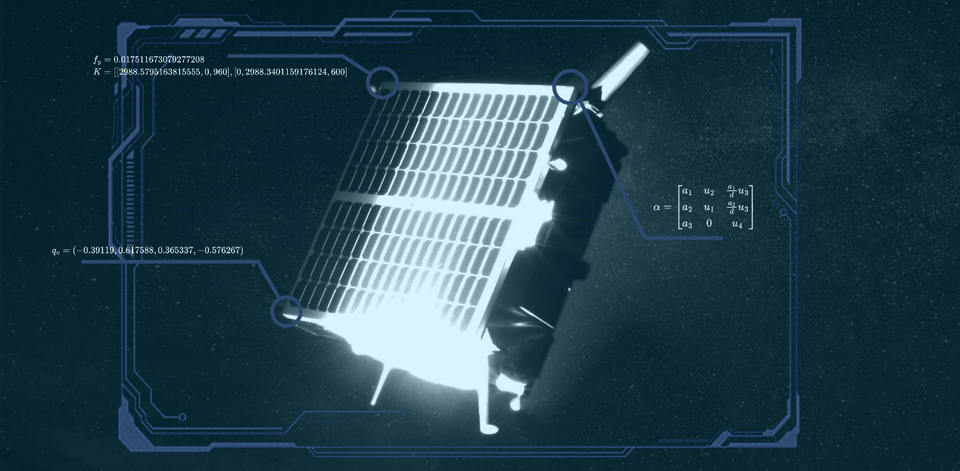
“It is a follow-on from our previous collaboration with Stanford University, focused on the same topic of ‘pose estimation,’” explains ESA Internal Research Fellow Gurvan Lecuyer. “This is the ability to identify the relative position and pointing direction of a target satellite from single images of it moving through space.”
ESA software engineer Marcus Märtens adds: “Pose estimation is of high interest to machine vision researchers in general – for instance in terms of robotic hands trying to safely pick up packages, self-driving cars or drones – but is especially crucial for space; success would form the basis of autonomous spacecraft rendezvous, docking and servicing. The challenge is simple in scope, to perform accurate estimates using simply raw pixels from a single monochrome camera, representative of smaller low-cost missions lacking extra hardware such as radar, lidar or stereoscopic imagers.”
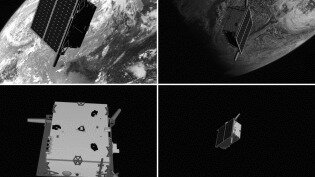
This latest challenge is open to machine learning groups and the aerospace community around the world. Participating teams will receive nearly 60 000 computer-generated images of a satellite in different configurations in space, seen variously against a background of Earth or starry deep space.
These synthetic images will be used to train the AIs to identify the pose of a mockup satellite in 9 531 unlabelled ‘Hardware-In-the-Loop’ (HIL) images, which have been captured with a real camera in a high-fidelity simulated space environment in the SLAB’s robotic facility.
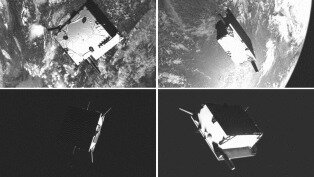
“The facility is carefully calibrated to estimate the accurate pose labels for each image samples down to a scale of millimeters at close range and a fraction of a degree,” says Tae Ha 'Jeff' Park, the PhD student leading the project under the supervision of Simone D’Amico, Founder and Director of SLAB. “This allows a more accurate evaluation of an AI model’s performance on real images.”
“We have equipped our facility with lighting equipment to create space-like conditions, which can pose a real challenge for cameras,” explains Prof. D’Amico.
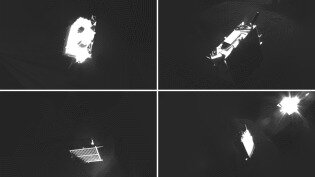
“In the vacuum of space, for instance, the contrast between light and dark is always very high, as with shaded craters on the Moon. Unfiltered sunlight can create intense reflection on satellite surfaces, blurring views, while at the same time the reflected glow of Earth gives rise to diffuse lighting. Using images taken in such conditions, we want to see how well AIs trained on synthetic images will be able to cope with real-world images like these – often termed the ‘domain gap transfer’ problem. We need to be sure that the computations involved would actually work in space.”
Like its predecessor competition from two years ago, this contest takes an actual space mission as a starting point, for maximum realism: the double-satellite PRISMA mission – launched by the then Swedish Space Corporation (now OHB Sweden) with the support of the German Aerospace Center DLR, the Technical University of Denmark and French space agency CNES – launched in 2010. Its two small satellites, Tango and Mango, took images of each other as they flew through space.
Prof. D’Amico has direct experience in several satellite formation-flying and rendezvous missions, including PRISMA, having worked on its relative navigation and control systems and serving as its Principal Investigator for DLR.
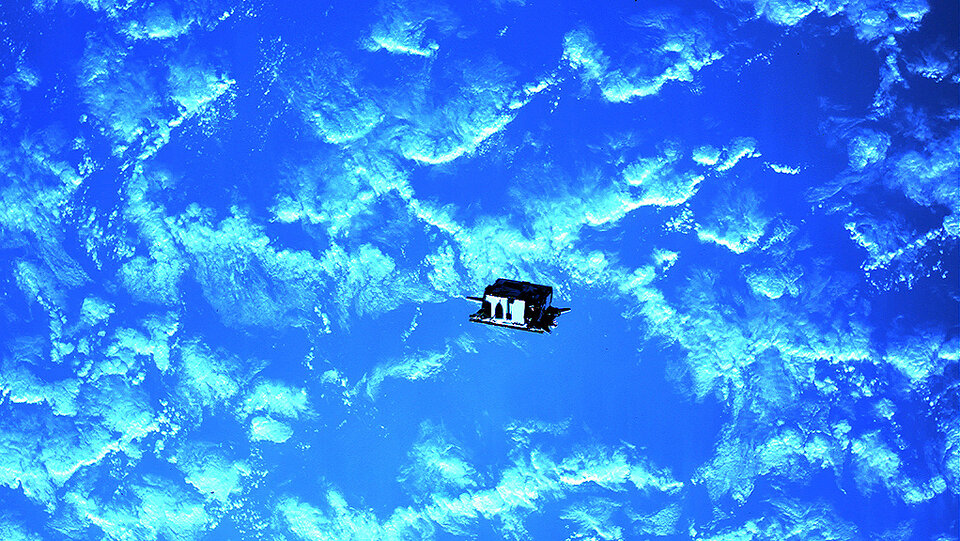
The original pose estimation competition relied mainly on synthetic images with only a minimum amount of HIL images from the robotic testbed. However, the dataset proved sufficiently popular to machine learning researchers that the competition was made open-ended, with more than 70 teams participating worldwide, producing nearly a thousand submissions.
“The results basically pushed forward the state of the art,” comments Dario Izzo of ESA’s ACT. “We achieved error levels of an order of magnitude less than before. But the competition was not sufficient to really have a comprehensive study of the applicability of these solutions to the real world – the poses were limited, and far from covering every possible configuration. So this time we combine an increased number of images with a much greater variety of poses, as well as realistic lighting conditions.”
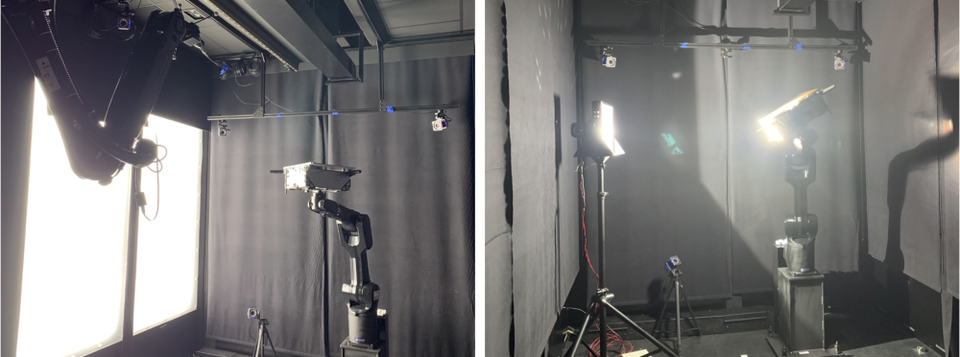
This new Spacecraft PosE Estimation Dataset (SPEED+) challenge is the latest machine learning competition hosted at an updated version of ACT’s Kelvins website, named after the temperature unit of measurement – with the idea that competitors should aim to reach the lowest possible error, as close as possible to absolute zero. The updated website includes new features for community building and discussions among participants. The competition is due to start on 25 October. Click here to find out how to get involved.