Bayesian Inference for Asteroid Selection
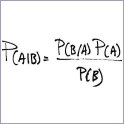
Can we teach a machine to beat a human expert in solving the asteroid selection problem?
Many space missions have the goal of better studying and understanding the chemical and physical characterization of asteroids and comets in order to make us know more on the formation of the Solar system. Past missions to asteroids are, among others, GIOTTO [1] and Deep Impact [2]. The future mission DAWN, to be launched in June 2007, will travel to the two oldest and most massive asteroids in our solar system: Vesta and Ceres. In such a mission, given a set of scientific requirements, such as the sequential visit to n asteroids, each of them having some predefined characteristics, the misssion analyst is asked to find the best selection of asteroids according to some criteria such as the minimal fuel consumption (the objective function J). However, optimal asteroid selection is not trivial. The international scientific community has been competing this year on a similar problem during the 2nd edition of the Global Trajectory Optimization organized by JPL.
The ACT is tackling the challange of employing Bayesian techniques for infering the optimal asteroid sequence. Given an initial space of randomly generated solutions, a Bayesian network is trained on the asteroid orbital paramters in order to find conditions on these parameters that would "probably" lead to high values of the objective function J. These conditions are used as a pruning mechanism to create better random sequences that will be fed to the Bayesian network to improve its performance. This dynamic process is iterated, until high values of the probability distributions of the orbital parameters are observed.