Artificial intelligence in space
Artificial intelligence. We hear more and more about it. Some promise that it will transform society for the better, others claim it is dangerous. How are we ensuring that it is used effectively and safely in space?
What is artificial intelligence?
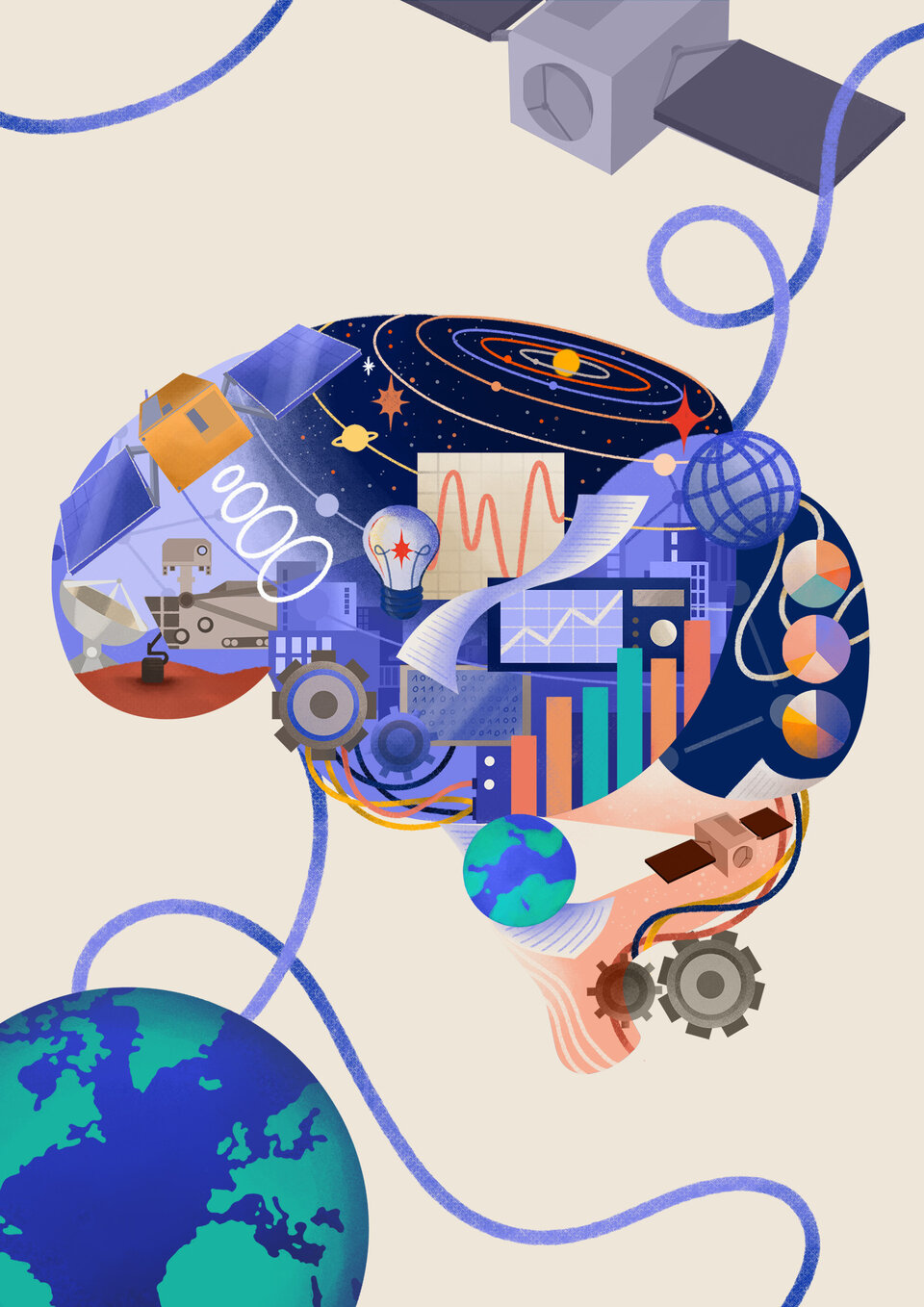
AI refers to the ability of a computer or robot to mimic human intelligence. It could cover, for example, computers that analyse data independently or the autonomous systems embedded in driverless vehicles.
Nowadays, it is common for AI to be achieved through machine learning. Machine learning teaches machines to learn for themselves. It is a way of ‘training’ a relatively simple algorithm to become more complex. Huge amounts of data are fed into the algorithm, which adjusts and improves itself over time.
Deep learning is a specialised technique within machine learning, whereby a machine uses multi-layered artificial neural networks to train itself on complex tasks like image recognition. This can happen via supervised learning (for example, feeding a system photos of the Moon and Earth until it can successfully identify both) or unsupervised learning, where the network finds structure by itself. Examples of deep learning are online translation services, chatbots and navigation systems.

We are already beginning to see AI implemented into new space technologies. It is used to control large satellite constellations, to analyse the huge amounts of data that satellites collect, and to process data directly onboard satellites.
But there is potential to go further. And that is why ESA, including the Discovery & Preparation elements of the Agency’s Basic Activities, is exploring new ways that AI can make all areas of space – from Earth observation to satellite navigation – more effective.
What is Discovery & Preparation doing in this area?
In 2022, ESA Discovery funded 12 projects that explored whether we can apply the latest developments in AI and advanced computing paradigms to make satellites more reactive, agile and autonomous. They were selected through the Open Space Innovation Platform ‘Cognitive cloud computing in space’ call for ideas. The hope was to generate new practical applications that support life on Earth and our exploration of other planets.
Some of the projects researched how AI-equipped satellites could directly improve our lives on Earth, for example by more effectively detecting methane leaks and managing disasters from space. Others looked at how these smarter satellites could support more sustainable exploration of the Moon and make lunar rovers more independent. Find out more and discover the full list of projects in our dedicated article.
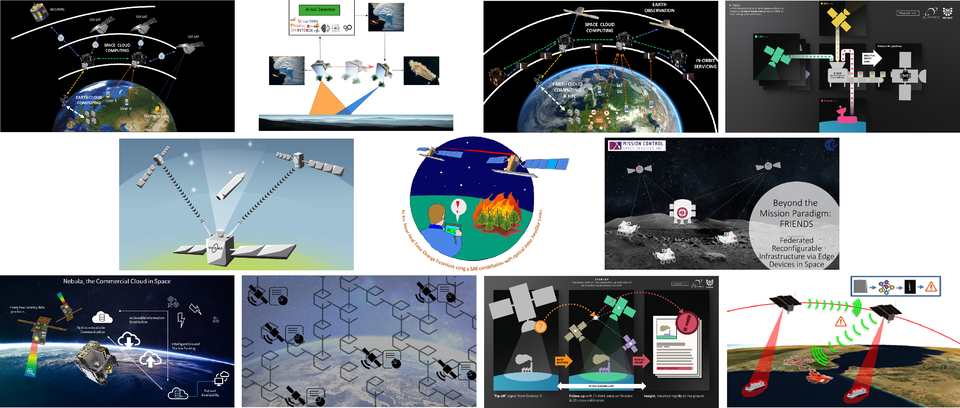
Several of the cognitive computing projects focused on constellations of satellites, which we are seeing more and more of in our modern world. These are complicated to operate and require regular collision avoidance manoeuvres. Discovery was already looking into automating constellations in the year 2000, for example by enabling autonomous navigation, telemetry analysis and software upgrades. A more recent study developed the idea further, focusing on the autonomous management of complex constellations to reduce the workload of ground operators. Other Discovery studies investigated how a swarm of tiny satellites can evolve a collective consciousness, and looked into how AI can be used in advanced mission operations and technologies, as well as in innovative security concepts, mechanisms and architectures.
Also in 2022, Discovery kicked off a number of projects to develop software, concepts and protocols to push ESA’s experimental OPS-SAT space lab to its limits. Several of these included AI. OPS-SAT spacecraft manager David Evans said that “AI is a broad term for an effectively infinite toolbox, and it was great to see concrete applications using a variety of tools from across the field”.
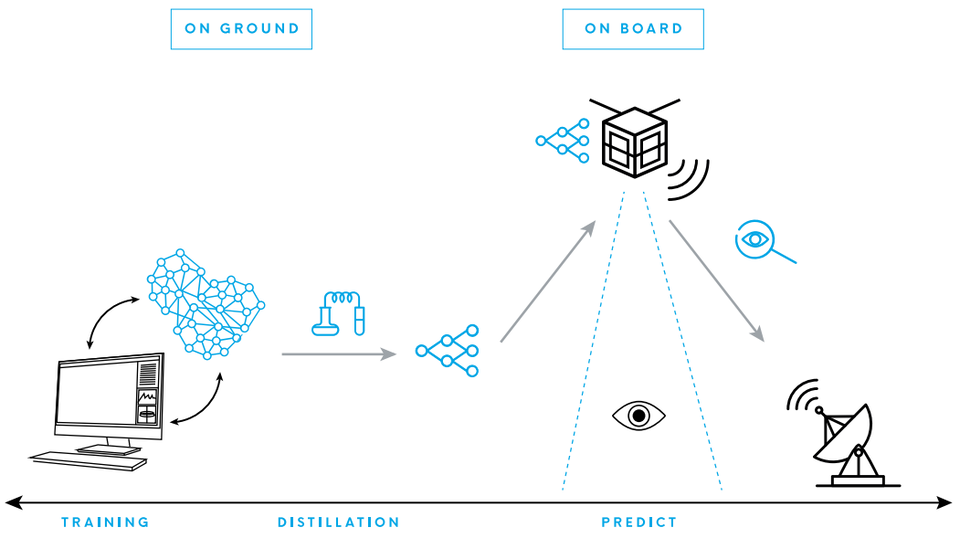
The OPS-SAT projects using AI included running deep learning algorithms to improve the spacecraft’s image quality, unlocking new methods to employ deep learning on the spacecraft, detecting and tracking features on Earth’s surface, using an AI technique called ‘reinforcement learning’ to better control the orientation of the spacecraft, and detecting forests using deep learning.
The previous year, Discovery provided funding to 26 projects working on detecting and tracking marine litter using satellites. Several of these made use of AI, for example by training AI models to detect certain types of plastic, using AI to identify floating plastic particles, and combining AI with drones to automatically detect submerged plastic litter.
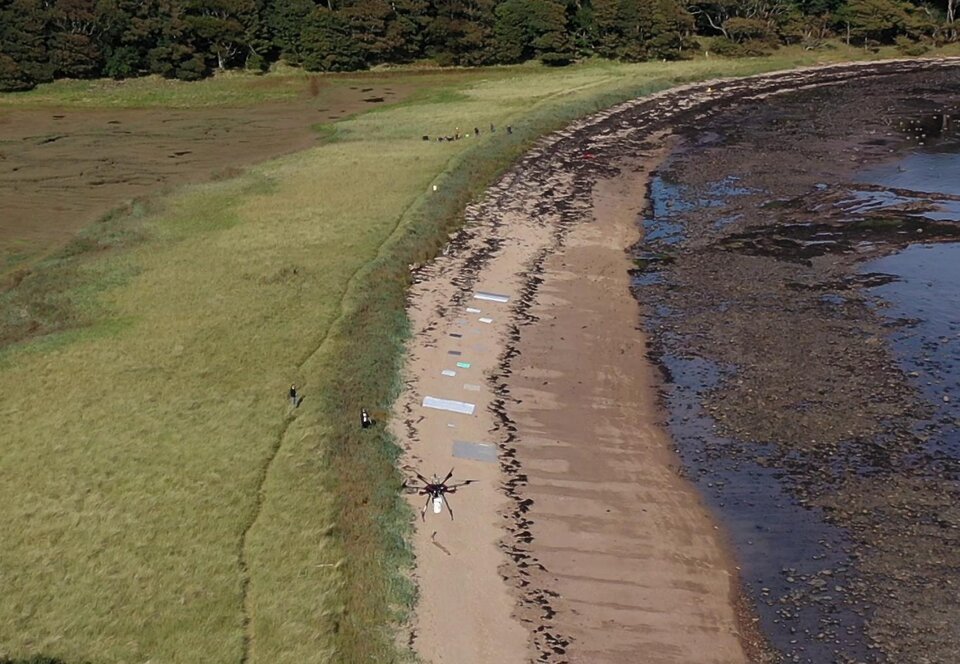
Autonomous navigation is a key application of AI, that would help us navigate around Earth and other planets. One Discovery study explored using AI to help spacecraft navigate independently in unknown environments. ESA’s Advanced Concepts Team (ACT) also investigated using machine learning in the area of guidance, navigation and control. In particular, they looked into using big swarms of small robots that share their information in a network: if one robot learns from experience that a certain manoeuvre is beneficial, the whole swarm learns this. This is called hive learning.
Tasked with preparing ESA for disruptive change, the ACT has worked on various other projects related to AI, including assessing brain-inspired technologies for space applications, using AI to map the geometry and gravity of space rocks, as well as to develop novel materials.
Huge progress has also been made recently in AI-based natural language processing, mainly driven by chatbots and vocal home assistants. A Discovery study spun in these technologies into space engineering processes, studying how natural language processing can help space engineers in their daily activities.
ESA-wide applications of AI/ML
Going from this exploratory research to real space applications may seem like a big step but ESA is already starting to use AI in its space missions. For example, rovers can autonomously navigate around obstacles and the download of data from Mars rovers is being scheduled using AI. Artificial intelligence is also aiding astronauts on board the International Space Station (ISS), more details of which can be found in the next section of this article.
ESA’s Hera planetary defence mission will make use of AI as it steers itself through space towards an asteroid, taking a similar approach to self-driving cars. Whilst most deep-space missions have a definitive driver back on Earth, Hera will fuse data from different sensors to build up a model of its surroundings and make decisions onboard, all autonomously.
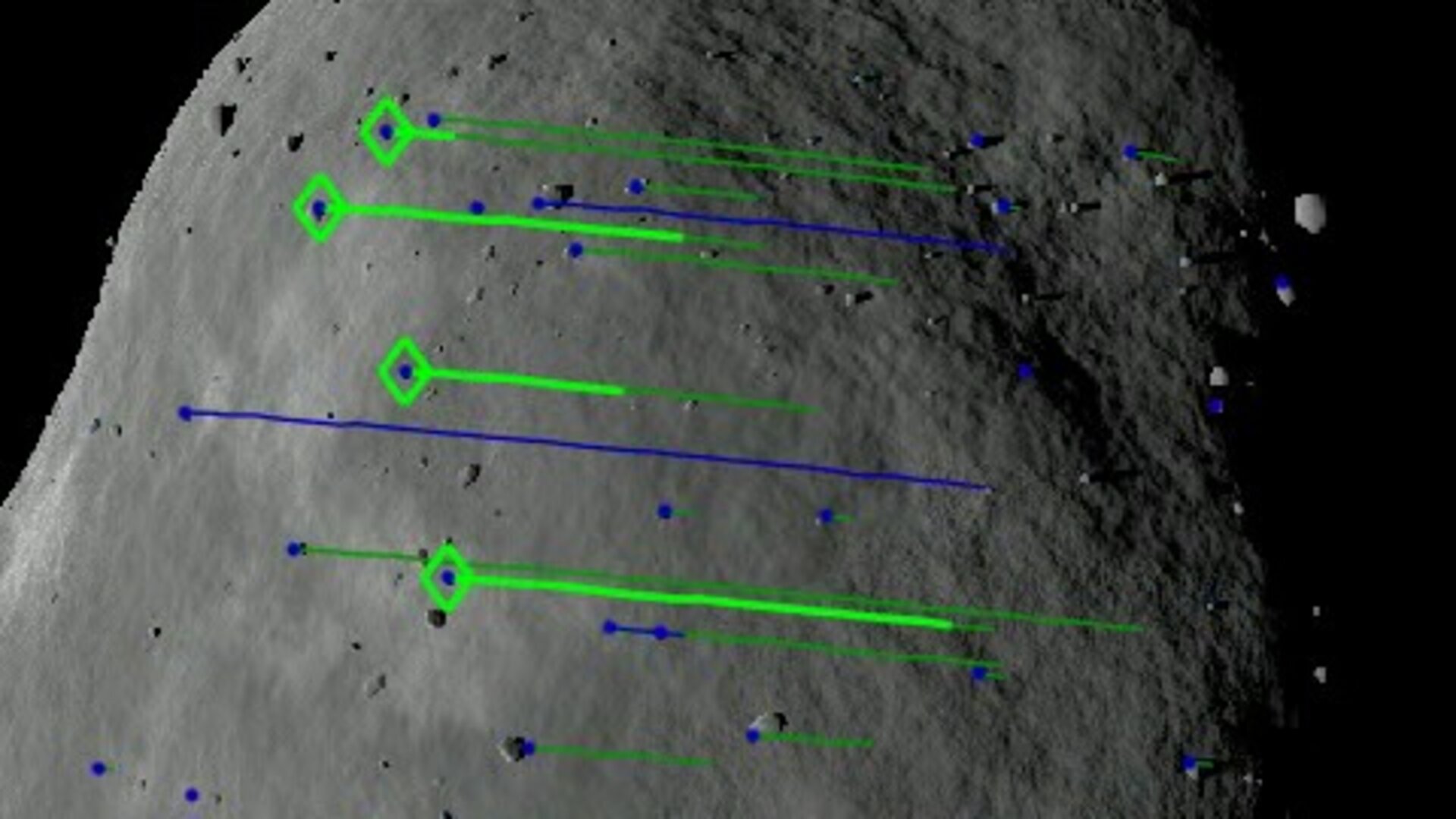
Access the video
Satellites orbiting Earth also require more autonomy, as they need to make more frequent collision avoidance manoeuvres to evade increasing amounts of space debris. In January 2021, ESA and the German Research Center for Artificial Intelligence (DFKI) established ESA_Lab@DFKI, a technology transfer lab that works on AI systems for satellite autonomy, collision avoidance capabilities and more.
Furthermore, ESA has gained ample experience using AI to plough through enormous amounts of data to extract meaningful information. This technique has already been implemented in more ‘Earthly’ applications, including monitoring the number of cars at a shopping centre, predicting retailers' financial performances, monitoring climate change and supporting police forces in their efforts to catch perpetrators.
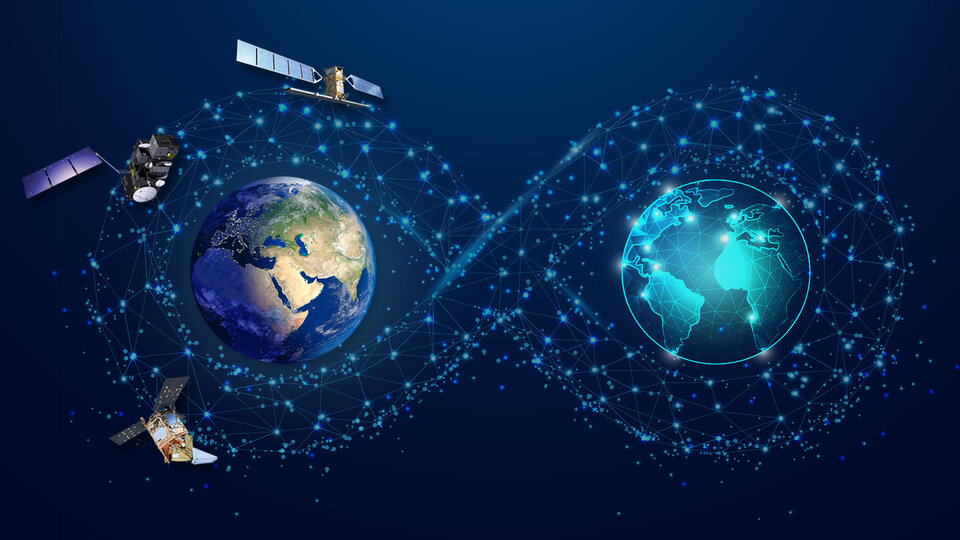
Earth observation is one area where AI is already being used more extensively. ESA is currently working towards a Digital Twin of Earth, a replica constantly fed with Earth observation data and AI to help visualise and forecast natural and human activity on the planet.
And the Agency’s ‘Rapid Action Earth observation’ dashboard is showing how AI can be used to monitor economic indicators – for example, the combination of commercial satellite data and AI has been used to monitor production changes at a car manufacturer in Germany and plane traffic at Barcelona airport. Moreover, FSSCat – launched in September 2020 – is the first European Earth observation mission to carry AI on board, in the form of the ɸ-sat-1 AI chip. By filtering data onboard, ɸ-sat-1 is improving the efficiency of sending vast quantities of data back to Earth.
AI is also being applied to satellite navigation, where it can improve space and Earth weather forecasting, and help identify rogue drones in sensitive airspace. AI-equipped navigation an enhance the performance of autonomous cars and boats; an ESA-led project applied AI to achieve autonomous situational awareness, enabling a ship to reliably sense its own environment.
What are other space agencies doing in this area?
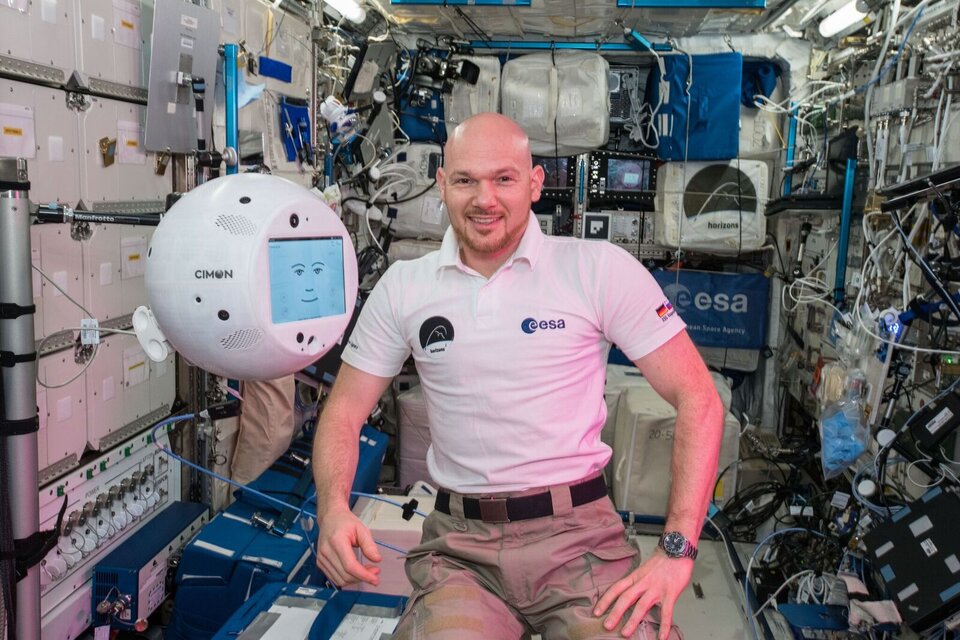
The German Aerospace Center (DLR) has been developing AI methods for space and Earth applications for many years and in 2021 set up an Institute of Artificial Intelligence Security. In 2018 DLR launched an AI assistant to support its astronauts in their daily tasks onboard the ISS. Fully voice-controlled CIMON (Crew Interactive MObile companioN) is able to see, speak, hear, understand and even fly! CIMON returned after 14 months, but CIMON-2 arrived in December 2019 to replace it.
NASA is also using AI for many applications, and has set up an Artificial Intelligence Group that performs basic research that supports scientific analysis, spacecraft operations, mission analysis, deep space network operations and space transportation systems. The Agency investigated making communication networks more efficient and reliable using cognitive radio, which picks out the ‘white noise’ areas in communication bands and uses them to transmit data; this maximises the use of the limited telecommunication bands available and minimises delay times. It also applied AI to calibrate images of the Sun, improving the data that scientists use for solar research. For deep-space exploration NASA has also looked into designing more autonomous spacecraft and landers, so that decisions can be taken on site, removing the delay resulting from communication relay times.
NASA cooperated with Google to train its extensive AI algorithms to effectively sift through the data from the Kepler exoplanet mission to look for signals from an exoplanet crossing in front of its parent star. This successful collaboration quickly led to the discovery of two new exoplanets previously missed by human scientists. Following its success, AI has been used on data from NASA’s TESS mission to identify candidate exoplanets.

The Japanese space agency’s (JAXA) Epsilon rocket was the first in history to incorporate artificial intelligence; by performing checks and monitoring its performance autonomously, Epsilon makes launching a payload into space simpler than ever before. JAXA also developed an intelligent robot called ‘Int-ball’ that takes pictures of experiments in the ISS, saving astronauts’ valuable time.
Meanwhile, French space agency CNES worked with French company Clemessy to optimise the filling of rocket tanks using AI neural networks, the UK Space Agency funded a project that uses AI to detect buried archaeological remains in satellite imagery, and the Italian Space Agency even co-founded an AI-focused company.
Last updated 3 August 2023.